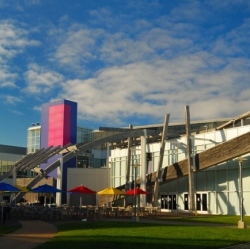
Google released neural network-powered voice recognition in 2011. Now, it’s announced that the addition of recurrent neural networks will make the tech much faster and more accurate. The Google Speech Team explains that it’s added Connectionist Temporal Classification and sequence discriminative training techniques to its algorithms.
If that doesn’t make much sense to you, here’s a straightforward explanation of how it works: In a traditional speech recogniser, the waveform spoken by a user is split into small consecutive slices or “frames” of 10 milliseconds of audio. Each frame is analysed for its frequency content, and the resulting feature vector is passed through an acoustic model… The recogniser then reconciles all this information to determine the sentence the user is speaking. If the user speaks the word “museum” for example – /m j u z i @ m/ in phonetic notation – it may be hard to tell where the /j/ sound ends and where the /u/ starts, but in truth the recogniser doesn’t care where exactly that transition happens: All it cares about is that these sounds were spoken.
Our improved acoustic models rely on Recurrent Neural Networks (RNN). RNNs have feedback loops in their topology, allowing them to model temporal dependencies: when the user speaks /u/ in the previous example, their articulatory apparatus is coming from a /j/ sound and from an /m/ sound before. Try saying it out loud – “museum” – it flows very naturally in one breath, and RNNs can capture that.
By introducing that ability to include information about sounds on either side of each snippet, the algorithms stands a far better chance of understanding what you say. In fact, Google claims that it makes voice search far more accurate, particularly in noisy environments, as well as helping to make it “blazingly fast”.
You don’t even need to do anything to take advantage of the improvement: The new neural network approach is already being used by the Google search app for iOS and Android.