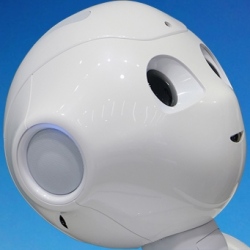
Humans have gained a lot of value by organizing all their knowledge and making it widely accessible, in textbooks, libraries, Wikipedia, and YouTube, to name a few examples. These pools of knowledge aren’t valuable just for grand scientific ventures but also for the trivial stuff of everyday human lives.
However, the organized collections of knowledge that work for humans aren’t so great for robots. A robot wouldn’t get much useful information if it queried a search engine for how to “bring sweet tea from the kitchen.” Robots require something different, access to finer details for planning, control, and natural language understanding.
When asked to bring sweet tea, the robot would need access to the knowledge for interpreting the language symbols (“tea”) in terms of physical entities (“a particular container having sweet tea”), the spatial knowledge that sweet tea can be either on a table or in a fridge, and the knowledge for inferring how to grasp and manipulate objects. It’s possible to manually script a demo for one particular situation, but handling this across different tasks and in different environments is still an open problem.
In 2014, I started a project called RoboBrain at Cornell University along with PhD students Ashesh Jain and Ozan Sener. We now have collaborators at Stanford and Brown. What we’re working on is a way of sharing information that allows robots to gather whatever knowledge they need for a task. If one robot learns, then the knowledge is propagated to all the robots.
RoboBrain achieves this by gathering the knowledge from a variety of sources. The system stores multiple kinds of information, including symbols, natural language, visual or shape features, haptic properties, and motions.
This approach represents a huge shift in thinking. Historically, research groups working with robots have trained their robots in isolation. Yes, we often share ideas through publications and software that can be used by another research group, but what one robot might learn hasn’t been accessible to another researcher’s robot.
To add to the problem, research groups have been working on different problems, one might have focused on the computer vision problem of identifying a cup, while another worked on the language problem of what is a “cup,” while a third tackled how to grasp a cup.
That’s the kind of approach we need to get past. A cup is one object, not three. And a robot, just like a person, needs to be able to have all the knowledge it needs in one place.