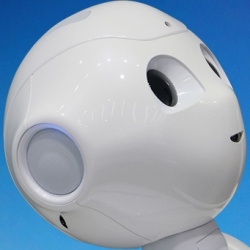
AI seems to be on fire, with tons of consumer demand and ample investor interest. VC investment in AI startups rose from $3.2 billion in 2014 to more than $9.5 billion in just the first five months of 2017. Prospects for AI include applications for health care, and other realms of technology, but AI isn’t a runaway train just yet.
The biggest hurdles AI has plenty of excitement backing it, but a few significant hurdles are keeping it from even more explosive growth:
1. Flexibility
One of the greatest advantages of young startups is their flexibility; big companies often suffer from protracted decision-making and an inability to pivot, but smaller, nimbler companies can react quickly and more efficiently to new circumstances.
However, AI startups don’t necessarily enjoy this advantage because AI is so complicated, and depends on so many unknown variables, that it’s hard to shift gears in the middle of a project. This can leave some AI startups dead in the water, or delay projects far past their original timelines.
2. Talent shortages
The number of professionals well-versed in machine learning and innovative enough to create new features is very small. There’s a talent shortage in AI, and it’s having profound effects on the pace of development in the industry. Proficient AI developers can demand huge salaries, making it hard for startups to afford them, and even startups with enough cash may struggle to fill their AI positions.
3. Competition
There are hundreds of interesting AI startups on the horizon or in the middle of development. That sounds like an exciting prospect for consumers hoping to get their hands on some next-generation technology, but it also presents an important problem: competition. Startups are forced to make faster decisions, go to market faster, and trim features in order to beat their competitors. This is causing some startups to burn out faster and others to launch with inferior products.
4. Sales cycle unpredictability
Few AI products have a clearly defined sales cycle at this point. For one thing, AI is still a relatively new field, so its market isn’t clearly defined. Many applications could do well to target either individual users or companies, and it’s hard to predict exactly what your product will look like at the end of its development cycle — even if you have a visionary plan in place. This makes it difficult for AI startups to predict their revenue streams accurately, and even more difficult to ensure enough revenue to stay afloat during their early stages of growth.
5. Machine learning complexity
It should go without saying that programming advanced AI features is ridiculously complicated. If you follow existing formulas and rely on the collective knowledge that we already have, machine learning becomes a simple matter of copying, pasting, and then tweaking. But to truly innovate in this field, you need a wealth of knowledge and experience, along with the spirit to try new things.
6. Processing power
Most AI systems demand huge amounts of processing power to work. Until recently, this has been a significant limiting factor; many startups didn’t have access to the processing units necessary to get the job done. Now, companies like Nvidia are enjoying the demand for processing chips for AI applications, and reporting record sales and interest. However, in general, processing growth hasn’t been able to fully keep up with the latest AI technology, and that fundamental limit may continue to be a problem for developers.
Will AI see a slowdown?
So is it likely we’ll see a slowdown in the advancement of AI technology? In the short-term, it’s possible, though the high levels of consumer excitement and venture capital available will likely counteract some of these effects. As the tech becomes more accessible and easier to grasp, innovators in the field will naturally overcome many of these problems. Until then, they’ll continue complicating an already complicated industry.