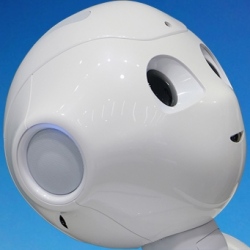
With great power comes great responsibility, and artificial-intelligence technology is getting much more powerful. Companies in the vanguard of developing and deploying machine learning and AI are now starting to talk openly about ethical challenges raised by their increasingly smart creations.
“We’re here at an inflection point for AI,” said Eric Horvitz, managing director of Microsoft Research, at MIT Technology Review’s EmTech conference this week. “We have an ethical imperative to harness AI to protect and preserve over time.”
Horvitz spoke alongside researchers from IBM and Google pondering similar issues. One shared concern was that recent advances are leading companies to put software in positions with very direct control over humans,for example in health care.
Francesca Rossi, a researcher at IBM, gave the example of a machine providing assistance or companionship to elderly people. “This robot will have to follow cultural norms that are culture-specific and task-specific,” she said. “[And] if you were to deploy in the U.S. or Japan, that behavior would have to be very different.”
Such robots may still be a ways off, but ethical challenges raised by AI are already here. As businesses and governments rely more on machine-learning systems to make decisions, blind spots or biases in the technology can effectively lead to discrimination against certain types of people.
A ProPublica investigation last year, for example, found that a risk scoring system used in some states to inform criminal sentencing was biased against blacks. Similarly, Horvitz described how an emotion recognition service developed at Microsoft for use by businesses was initially inaccurate for small children, because it was trained using a grab bag of photos that wasn’t properly curated.
Maya Gupta, a researcher at Google, called for the industry to work harder on developing processes to ensure data used to train algorithms isn’t skewed. “A lot of times these data sets are being created in a somewhat automated way,” said Gupta. “We have to think more about are we sampling enough from minority groups to be sure we did a good enough job?”
In the past year, many efforts to research the ethical challenges of machine learning and AI have sprung up in academia and industry. The University of California, Berkeley; Harvard; and the Universities of Oxford and Cambridge have all started programs or institutes to work on ethics and safety in AI. In 2016, Amazon, Microsoft, Google, IBM, and Facebook jointly founded a nonprofit called Partnership on AI to work on the problem (Apple joined in January).
Companies are also taking individual action to build safeguards around their technology. Gupta highlighted research at Google that is testing ways to correct biased machine-learning models, or prevent them from becoming skewed in the first place. Horvitz described Microsoft’s internal ethics board for AI, dubbed AETHER, which considers things like new decision algorithms developed for the company’s in-cloud services. Although currently populated with Microsoft employees, in future the company hopes to add outside voices as well. Google has started its own AI ethics board.
Perhaps unsurprisingly, the companies creating such programs generally argue they obviate the need for new forms of government regulation of artificial intelligence. But at EmTech, Horvitz also encouraged discussion of extreme outcomes that might lead some people to conclude the opposite.
In February he convened a workshop where experts laid out in detail how AI might harm society by doing things like messing with the stock market or election results. “If you’re proactive, you can come up with outcomes that are feasible and put mechanisms in place to disrupt them now,” said Horvitz.
That kind of talk seemed to unnerve some of those he shared the stage with in San Francisco. Gupta of Google encouraged people to also consider how taking some decisions out of the hands of humans could make the world more moral than it is now.
“Machine learning is controllable and precise and measurable with statistics,” she said. “There are so many possibilities for more fairness, and more ethical results.”