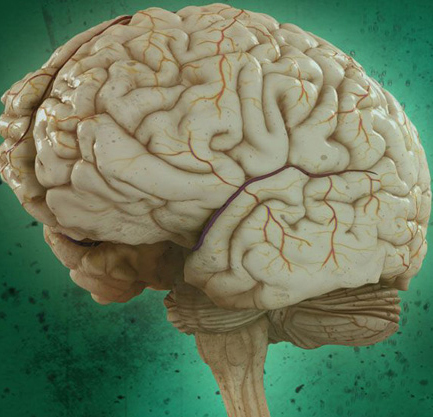
ChatGPT, DALL-E, Stable Diffusion, and other generative AIs have taken the world by storm. They create fabulous poetry and images. They’re seeping into every nook of our world, from marketing to writing legal briefs and drug discovery. They seem like the poster child for a man-machine mind meld success story.
But under the hood, things are looking less peachy. These systems are massive energy hogs, requiring data centers that spit out thousands of tons of carbon emissions—further stressing an already volatile climate—and suck up billions of dollars. As the neural networks become more sophisticated and more widely used, energy consumption is likely to skyrocket even more.
Plenty of ink has been spilled on generative AI’s carbon footprint. Its energy demand could be its downfall, hindering development as it further grows. Using current hardware, generative AI is “expected to stall soon if it continues to rely on standard computing hardware,” said Dr. Hechen Wang at Intel Labs.
It’s high time we build sustainable AI.
This week, a study from IBM took a practical step in that direction. They created a 14-nanometer analog chip packed with 35 million memory units. Unlike current chips, computation happens directly within those units, nixing the need to shuttle data back and forth—in turn saving energy.
Data shuttling can increase energy consumption anywhere from 3 to 10,000 times above what’s required for the actual computation, said Wang.
The chip was highly efficient when challenged with two speech recognition tasks. One, Google Speech Commands, is small but practical. Here, speed is key. The other, Librispeech, is a mammoth system that helps transcribe speech to text, taxing the chip’s ability to process massive amounts of data.
When pitted against conventional computers, the chip performed equally as accurately but finished the job faster and with far less energy, using less than a tenth of what’s normally required for some tasks.
“These are, to our knowledge, the first demonstrations of commercially relevant accuracy levels on a commercially relevant model…with efficiency and massive parallelism” for an analog chip, the team said.
This is hardly the first analog chip. However, it pushes the idea of neuromorphic computing into the realm of practicality—a chip that could one day power your phone, smart home, and other devices with an efficiency near that of the brain.
Current computers are built on the Von Neumann architecture. Think of it as a house with multiple rooms. One, the central processing unit (CPU), analyzes data. Another stores memory.
For each calculation, the computer needs to shuttle data back and forth between those two rooms, and it takes time and energy and decreases efficiency.
The brain, in contrast, combines both computation and memory into a studio apartment. Its mushroom-like junctions, called synapses, both form neural networks and store memories at the same location. Synapses are highly flexible, adjusting how strongly they connect with other neurons based on stored memory and new learnings—a property called “weights.” Our brains quickly adapt to an ever-changing environment by adjusting these synaptic weights.
IBM has been at the forefront of designing analog chips that mimic brain computation. A breakthrough came in 2016, when they introduced a chip based on a fascinating material usually found in rewritable CDs. The material changes its physical state and shape-shifts from a goopy soup to crystal-like structures when zapped with electricity—akin to a digital 0 and 1.
Here’s the key: the chip can also exist in a hybrid state. In other words, similar to a biological synapse, the artificial one can encode a myriad of different weights—not just binary—allowing it to accumulate multiple calculations without having to move a single bit of data.