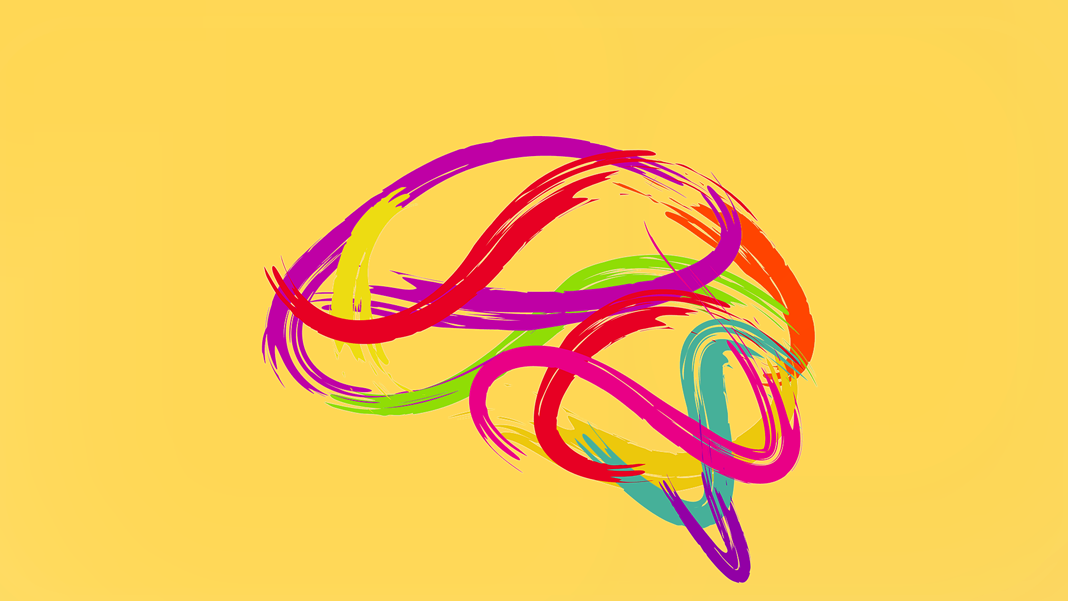
Compared to real human brains, AI algorithms are highly simplified, even “Cartoonish.” But can they teach us anything about how the brain works?
By studying how deep learning algorithms perform, we can distil high-level theories for the brain’s processes-inspirations to be further tested in the lab.
“AI [algorithms] have already been useful for understanding the brain even though they are not faithful models of physiology.” The key point, she said, is that they can provide representations-that is, an overall mathematical view of how neurons assemble into circuits to drive cognition, memory, and behaviour.
What are deep learning models missing? To panellist Dr. Cian O’Donnell at Ulster University, the answer is a lot.
Although we often talk about the brain as a biological computer, it runs on both electrical and chemical information. Incorporating molecular data into artificial neural networks could nudge AI closer to a biological brain, he argued.
Several computational strategies the brain uses aren’t yet used by deep learning.
Dubbed the “Little brain,” the cerebellum is usually known for its role in motion and balance. Enter GPT-3, a deep learning model with crazy language writing abilities.
In this highlighted study, led by Dr. Alexander Huth at the University of Texas, volunteers listened to hours of podcasts while getting their brains scanned with fMRI. The team next used these data to train AI models, based on five language features, that can predict how their brains fire up.
“[This] would not have been possible without deep neural networks. Despite being inspired by the brain, deep learning is only loosely based on the wiggly bio-hardware inside our heads.
AI is also not subject to biological constraints, allowing processing speeds that massively exceed that of human brains.
“The brain has multiple levels of organization,” going from genes and molecules to cells that connect into circuits, which magically lead to cognition and behaviour, he said.
It’s not hard to see biological aspects that aren’t in current deep learning models. Take astrocytes, a cell type in the brain that’s increasingly acknowledged for its role in learning.
As O’Donnell explains, three aspects could move deep learning towards higher biological probability: adding in biological details that underlie computation, learning, and physical constraints.
The brain learns in a dramatically different manner compared to deep learning algorithms. While deep neural networks are highly efficient at one job, the brain is flexible in many, all the time.
Deep learning has also traditionally relied on supervised learning-in that it requires many examples with the correct answer for training-while the brain’s main computational method is unsupervised and often based on rewards. Physical restraints in the brain could also contribute to its efficacy.
Finally, the brain minimizes space wastage: its input and output cables are like a highly mashed bowl of spaghetti, but their 3D position in the brain can influence their computation; neurons near each other can share a bath of chemical modulators, even when not directly connected.
This local but diffused regulation is something very much missing from deep learning models. These may seem like nitty-gritty details, but they could push the brain’s computational strategies toward different paths than deep learning.
Bottom line? “I do think the link between deep learning and brains is worth exploring, but there are lots of known and unknown biological mechanisms that aren’t typically incorporated into deep neural networks,” said O’Donnell.
Aspects of neuroscience conventionally not considered in deep learning-say, motivation or attention-are now increasingly popular among the deep learning crowd.
For Solla, the critical point is to keep deep learning models close-but not too close-to an actual brain.
All three panellists agreed that the next crux is figuring out the sweet spot between abstraction and neurobiological accuracy so that deep learning can form synergies with neuroscience.
“With deep learning networks you can train them to do well on tasks” that standard brain-inspired models couldn’t previously solve.