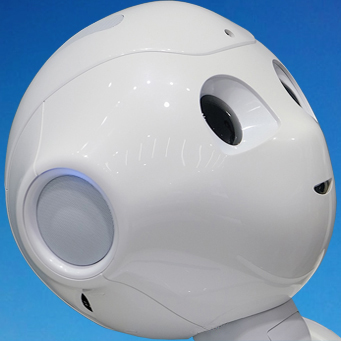
For all the talk about how artificial intelligence technology is transforming entire industries, the reality is that most businesses struggle to obtain real value from AI. 65% of organizations that have invested in AI in recent years haven’t yet seen any tangible gains from those investments, according to a 2019 survey conducted by MIT Sloan Management Review and the Boston Consulting Group.
A quarter of businesses implementing AI projects see at least 50% of those projects fail, with “Lack of skilled staff” and “Unrealistic expectations” among the top reasons for failure, per research from IDC. A major factor behind these struggles is the high algorithmic complexity of deep learning models.
Algorithmic complexity refers to the computational complexity of building and running these models in production.
Faced with prolonged development cycles, high computing costs, unsatisfying inference performance, and other challenges, developers often find themselves stuck in the development stage of AI adoption, attempting to perfect deep learning models through manual trial-and-error, and nowhere near the production stage.
Data scientists rely on facsimiles of other models, which ultimately prove to be poor fits for their unique business problems.
If human-developed algorithms inevitably run up against barriers of cost, time, manpower, and business fit, how can the AI industry break those barriers? The answer lies in algorithms that are designed by algorithms – a phenomenon that has been confined to academia to date but which will open up groundbreaking applications across industries when it is commercialized in the coming years.
Automating complex processes in the AI lifecycle will also make the benefits of AI more accessible, meaning it will be easier for organizations that lack large tech budgets and development staff to tap into the technology’s true transformative power.
Because the task of creating effective deep learning models has become too much of a challenge for humans to tackle alone, organizations clearly need a more efficient approach.
With data scientists regularly bogged down by deep learning’s algorithmic complexity, development teams have struggled to design solutions and have been forced to manually tweak and optimize models – an inefficient process that often comes at the expense of a product’s performance or quality.
Manually designing such models prolongs a product’s time-to-market exponentially.
AI practitioners require technologies that automate cumbersome processes involved in designing a deep learning model.
Similar to how Advanced Driver Assistance Systems are paving the way towards greater autonomy in the automotive industry, the AI industry needs its own technology to do the same.
AI building better AI. Encouragingly, AI is already being leveraged to simplify other tech-related tasks, like writing and reviewing code.
With innovative AI developers building on the work that’s already been done in this field, the exclusivity of AI technology set to give way to greater accessibility as the concept becomes more scalable and affordable in the coming years.