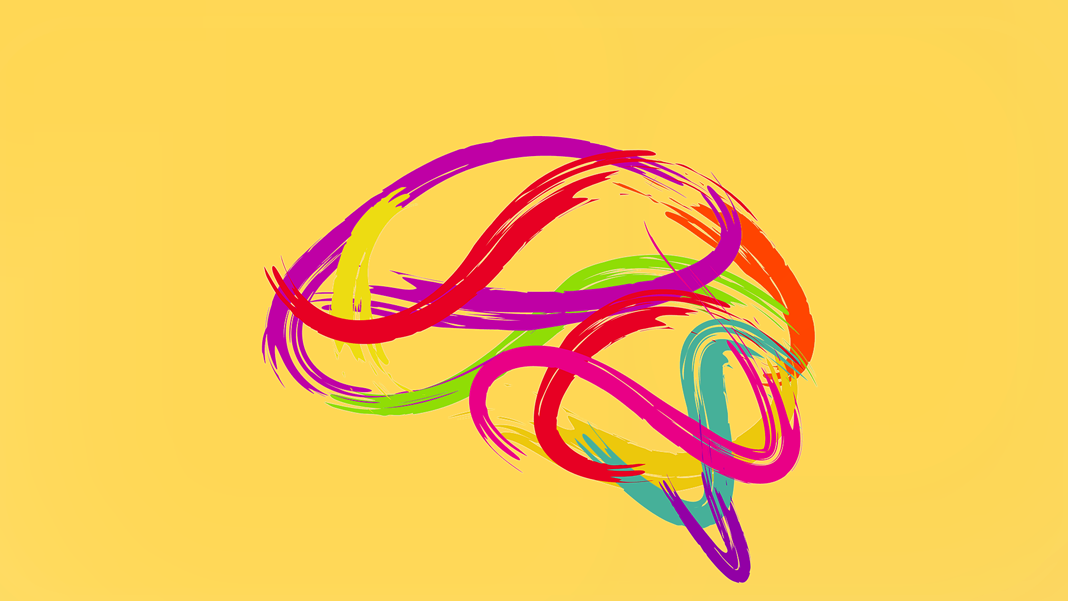
The human brain remains the most mysterious organ in our bodies. To some degree, researchers have succeeded in tapping into our thoughts and feelings, whether roughly grasping the content of our dreams or observing the impact of psilocybin on brain networks disrupted by depression. A study published earlier this year described a similar feat of decoding brain activity.
Ian Daly, a researcher from the University of Sussex in England, used brain scans to predict what piece of music people were listening to with 72 percent accuracy.
While participants in his study listened to music, Daly recorded their brain activity using both electroencephalography-which uses a network of electrodes and wires to pick up the electrical signals of neurons firing in the brain-and functional magnetic resonance imaging, which shows changes in blood oxygenation and flow that occur in response to neural activity.
EEG and fMRI have opposite strengths: the former is able to record brain activity over short periods of time, but only from the surface of the brain, since the electrodes sit on the scalp.
The latter can capture activity deeper in the brain, but only over longer periods of time.
He monitored the brain regions that had high activity during music trials versus no-music trials, pinpointing the left and right auditory cortex, the cerebellum, and the hippocampus as the critical regions for listening to music and having an emotional response to it-though he noted that there was a lot of variation between different participants in terms of the activity in each region.
Using both EEG and fMRI, Daly recorded brain activity from 18 people while they listened to 36 different songs.
He fed the brain activity data into a bi-directional long term short term deep neural network, creating a model that could reconstruct the music heard by participants using their EEG. A biLSTM is a type of recurrent neural network that’s commonly used for natural language processing applications.
Daly used the data from the biLSTM network to roughly reconstruct songs based on peoples’ EEG activity, and he was able to figure out which piece of music they’d been listening to with 72 percent accuracy.
Daly believes his method can be used to help develop brain-computer interfaces to assist people who’ve had a stroke or who suffer from other neurological conditions that can cause paralysis, such as ALS. BCIs that can translate brain activity into words would allow these people to communicate with their loved ones and care providers in a way that may otherwise be impossible.
While solutions already exist in the form of brain implants, if technology like Daly’s could accomplish similar outcomes, it would be much less invasive to patients.
“Thus, a neural decoding model that is able to reconstruct heard music from brain activity can form a reasonable step towards other forms of neural decoding models that have applications for aiding communication.”