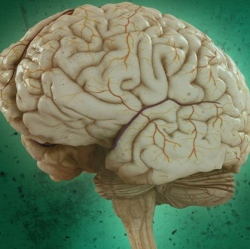
The researchers found that when trained for generic object recognition from natural photographs, several different DNNs developed visual representations that relate closely to human perceptual shape judgments, even though they were never explicitly trained for shape processing.
Deep neural networks are capable of learning to identify shapes, so “we’re on the right track in developing machines with a visual system and vocabulary as flexible and versatile as ours,” say KU Leuven researchers.
“For the first time, a dramatic increase in performance has been observed on object and scene categorization tasks, quickly reaching performance levels rivaling humans,” they note in an open-access paper in PLOS Computational Biology.
However, “We’re not there just yet,” say the researchers. “Even if machines will at some point be equipped with a visual system as powerful as ours, self-driving cars would still make occasional mistakes, although, unlike human drivers, they wouldn’t be distracted because they’re tired or busy texting. However, even in those rare instances when self-driving cars would err, their decisions would be at least as reasonable as ours.”