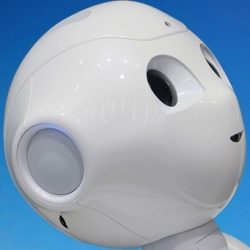
Video captioning has long been target for AI researchers. Now a team from Baidu and Perdue University have created a system that has achieved superior results using baseline datasets. Researchers working with recursive neural networks have made substantial gains in automatic video captioning.
The sentence generator produces simple short sentence that describes a specific short video intervals and a paragraph generator then captures the inter-sentence dependency and combines it with the paragraph history, and outputs a new overarching paragraph that describes the action in the video.
The work was done at Baidu and Perdue University, and has been published in a paper online.
The system was tested using nearly 2000 short YouTube segments. Each video clip has been annotated with multiple parallel sentences by different Open Turk contributors. The researchers aslo used another 185 longer videos from the dataset, TACoS-MultiLevel,
"The experiments show that our approach is able to generate multiple sentences or a paragraph for a long video and achieves the state-of-the-art results on two large-scale datasets," conclude the researchers.
Compared to other results with YouTube and TACos-MultiLevel videos, the approach used by the researchers outperformed the two baseline methods by large margins. "We expect to have even better performance if our object localization is improved," claim the study authors.
Captioning videos automatically has a lot of potential applications. The ability to generate text descriptions for unconstrained video is important because not only it is a critical step towards machine intelligence, but also it has many applications in daily scenarios such as video retrieval, automatic video subtitling, and for aids for the blind.
Even though the approach used in the study is able to produce paragraphs for video and has achieved encouraging results, it is still quite limited in application. The object detection routine has problems handling very small objects.
For instance it has a hard time when objects have similar shapes or appearances, like cucumbers and carrots or mangos and oranges. This is even more problematic when the objects are occluded, as is the case when a person is holding them.