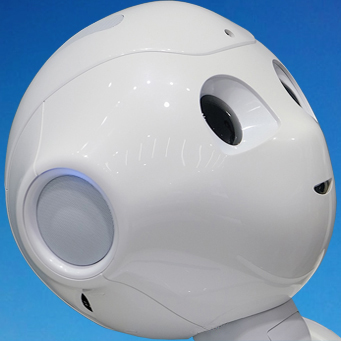
Deep reinforcement learning is having a superstar moment. Because the concept behind these algorithms is based on trial and error, a reinforcement learning AI “agent” only learns after being rewarded for its correct decisions. For complex problems, the time it takes an AI agent to try and fail to learn a solution can quickly become untenable.
Learning from trial and error comes intuitively to our brains. Reinforcement learning AI agents operate in a similar trial-and-error way. “Even in a moderately realistic environment, it may simply take too long to rationally respond to a given situation,” explained study author Dr. Hans Briegel at the Universität Innsbruck in Austria, who previously led efforts to speed up AI decision-making using quantum mechanics.
If there’s pressure that allows “only a certain time for a response, an agent may then be unable to cope with the situation and to learn at all,” he wrote.
Many attempts have tried speeding up reinforcement learning. Giving the AI agent a short-term “memory. In 2014, Briegel and colleagues showed that a “quantum brain” of sorts can help propel an AI agent’s decision-making process after learning.
Rather than building an entire reinforcement learning system using quantum mechanics, they turned to a hybrid approach that could prove to be more practical. At the heart of the quantum “trial” process is a quirk called superposition. Quantum mechanics is far weirder, in that photons can simultaneously be both 0 and 1, with a slightly different probability of “leaning towards” one or the other. This oddity is part of what makes quantum computing so powerful.
Take our reinforcement learning example of navigating a new campsite. In a quantum setup, however, the AI can turn left and right at the same time. So when searching for the correct path back to home base, the quantum system has a leg up in that it can simultaneously explore multiple routes, making it far faster than conventional, consecutive trail and error. “As a consequence, an agent that can explore its environment in superposition will learn significantly faster than its classical counterpart,” said Briegel.
Nanophotonic processors act kind of like our eyeglasses, which can carry out complex calculations that transform light that passes through them. For a light-based computer chip, it allows computation. The “error” or “reward” part of the new hardware comes from a classical computer. This setup, the team explains, allows them to more objectively judge any speed-ups in learning in real time.
In this way, a hybrid reinforcement learning agent alternates between quantum and classical computing, trying out ideas in wibbly-wobbly “multiverse” land while obtaining feedback in grounded, classic physics normality.
The quantum advantage blossoms when the task becomes more complex or difficult, allowing quantum mechanics to fully flex its superposition muscles. For these problems, the hybrid AI was 63 percent faster at learning a solution compared to traditional reinforcement learning, decreasing its learning effort from 270 guesses to 100. Now that scientists have shown a quantum boost for reinforcement learning speeds, the race for next-generation computing is even more lit. The partial-quantum setup could “aid specifically in problems where frequent search is needed, for example, network routing problems” that’s prevalent for a smooth-running internet, the authors wrote.
“We are just at the beginning of understanding the possibilities of quantum artificial intelligence,” said lead author Walther.