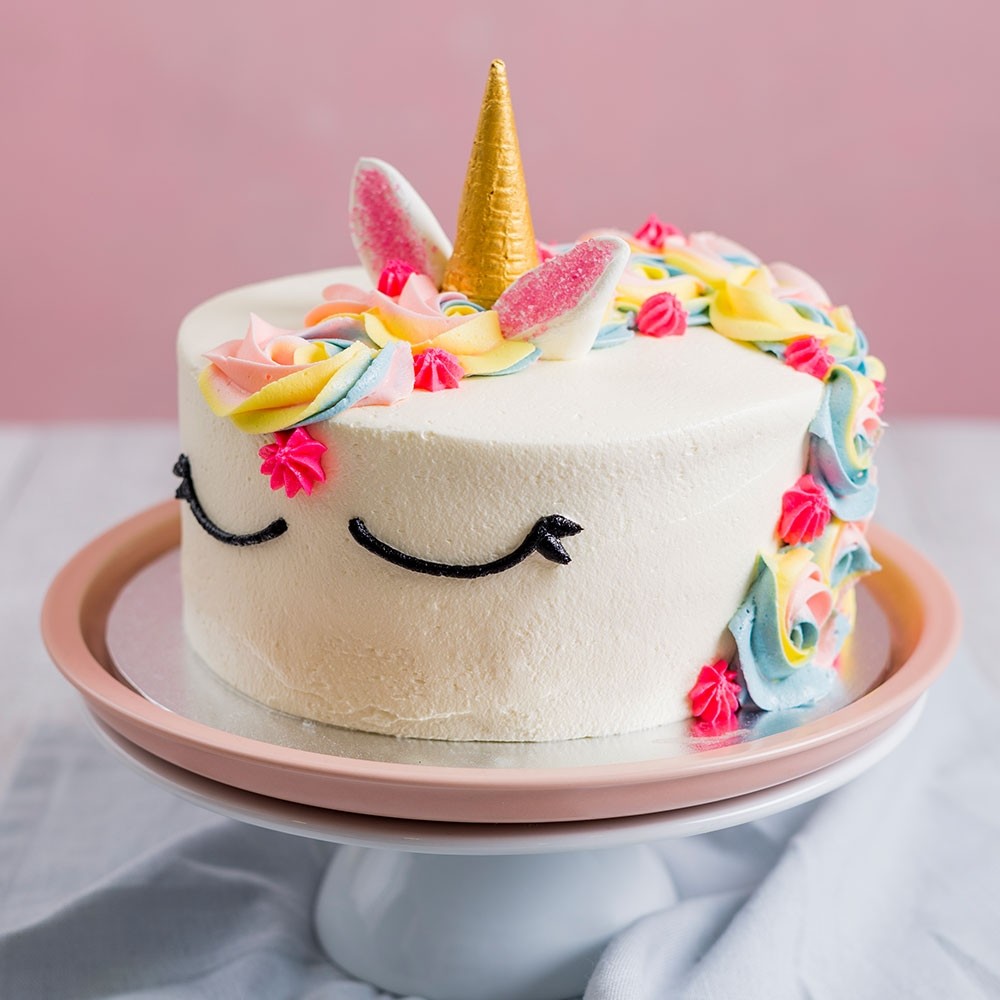
Robot gourmands are imminent, it seems, and they might someday craft recipes just as delicious as those from their human counterparts. In a newly published paper on the preprint server Arxiv.org (“KitcheNette: Predicting and Recommending Food Ingredient Pairings using Siamese Neural Networks“), scientists at Korea University describe an AI system that predicts ingredient pairings and ranks them by score. They say that it’s not only able to suggest complementary food pairings and to uncover novel ingredient combinations, but that it outperforms other baseline models by a wide margin.
“Many chefs, gourmets, and food-related researchers have focused on studying food pairing for decades,” wrote the paper’s coauthors. “Since food pairings are made based on the experiences of experts, food pairing itself is subjective and difficult to quantify. In this work, we introduce KitchenNette, which … predicts the scores of unknown pairings consisting of food ingredients that have infrequently or never been used in recipes.”
The team’s AI system consists of so-called Siamese networks, or two identical machine learning models that each take one of two data samples as inputs, along with a wide and deep system comprising wide linear models and deep neural networks. To train them, the scientists sourced a data set (Recipe1M) containing lists of ingredients and recipe instructions in text and images, from which they derived 356,451 known ingredient pairings and 3,567 unique ingredient names. A separate machine learning algorithm — Im2Recipe — extracted ingredient names, which the researchers used to compile a corpus of scores defining “complementary” food pairs of on a scale between “-1” and “1.”
To test the AI model’s culinary knowledge, the researchers chose three similar carbonated white wines — champagne, sparkling wine, and prosecco — and then calculated the score of each paired with a different ingredient. As might be expected, combinations like “champagne and orange twist” and “orange twist and sparkling wine” scored consistently high (0.33-0.45), while less orthodox pairings like “sparkling wine and onion” and “prosecco and onion” scored consistently low.
In another experiment, the coauthors found that the AI system generally recommended food ingredients used in everyday cooking and dining, like “tomato and lettuce,” “onion and ground beef,” and “pepper and oregano.” Perhaps more excitingly, it discovered novel food-drink combos that agreed with recommendations in The Flavor Bible, What to Drink with What You Eat, and other well-regarded gastronomic literature — for example, a variety of meat (e.g., beef, lamb) for red wine and authentic Japanese food ingredients to pair with sake.
The researchers leave to future work factoring in the chemical information about food ingredients and using more detailed information on food ingredients from encyclopedias, as well as using more “novel” and “authentic” recipes to help their model to recommend “more versatile” food ingredient pairings.
It’s worth noting that their work contributes to a growing body of AI recipe recommender systems. IBM recently announced that it’s teaming up with McCormick & Company to create new flavors and foods with machine learning. IBM’s Chef Watson, a research project that sought to create new recipes by analyzing the chemical composition of hundreds of different ingredients, produced more than 10,000 novel recipes. (A cookbook of its creations was published in 2015.) And New York startup Analytical Flavor Systems’ platform — Gastrograph — taps sensory data and machine learning algorithms to suss out products’ flavor profiles and identify areas for improvement.
Meanwhile, Los Angeles-based Halla’s I/O platform uses AI to generate Netflix-like recommendations for grocery, restaurant, and food delivery apps and websites, in part by leveraging a database of restaurant dish, recipe, ingredient, and grocery item taste and flavor attributes. Others like Foodpairing, Plant Jammer, and Dishq offer proprietary recommendation systems that take into account personal preferences. There’s also Tastewise, a platform that combines artificial intelligence (AI), predictive analytics, computer vision, and natural language processing to suss out emerging culinary trends.