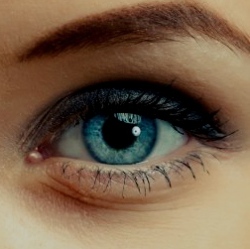
In a new paper, researchers at MIT have detailed a neural network capable of detecting depression. Unlike previous methods using machine learning, MIT’s neural net doesn’t require the subject to answer specific questions, it can make an assessment based on everyday conversation, or even just text.
"The first hints we have that a person is happy, excited, sad, or has some serious cognitive condition, such as depression, is through their speech," says lead author Tuka Alhanai in an MIT press release. "If you want to deploy models in scalable way … you want to minimize the amount of constraints you have on the data you’re using. You want to deploy it in any regular conversation and have the model pick up, from the natural interaction, the state of the individual."
The crux of the technology is its ability to apply what it’s learned from past subjects to new ones. The researchers used sequence modeling, a technique common in machine speech processing, to analyze both audio and text from a sample of 142 interactions. Crucially, only some of the individuals sampled were depressed. Gradually, the neural net was able to match certain words with certain patterns of speech.
"Words such as, say, sad, low, or down, may be paired with audio signals that are flatter and more monotone," explains the MIT release. "Individuals with depression may also speak slower and use longer pauses between words." The model then decides whether or not these patterns are truly indicative of depression, and if so, it knows to look for these patterns in other people.
Perhaps surprisingly, the model needs more data to work with to detect depression using audio samples than it does with text. In writing, it can identify depression from an average of seven questions and answers. But using audio samples, it needs about 30. The researchers suggest that this is because the patterns indicative of depression occur more quickly in text than in audio. Overall, the model can detect depression with 77 percent accuracy, though this figure is calculated from a number of metrics.
It’s hoped the technology could lead to the development of apps that could help people to monitor their own mental health using a mobile device, especially when living remotely and where cost, distance and time may hinder their seeing a clinician.
But the team thinks it could also be used to assist clinicians with their diagnoses in person. "Every patient will talk differently, and if the model sees changes maybe it will be a flag to the doctors," co-author James Glass explains in the same release. "This is a step forward in seeing if we can do something assistive to help clinicians."
As is often the case with neural networks, it seems there’s a significant challenge in understanding what, exactly, the model is doing. "Right now it’s a bit of a black box," Glass adds. "These systems, however, are more believable when you have an explanation of what they’re picking up. … The next challenge is finding out what data it’s seized upon."
The researchers think the model may also be useful for detecting other conditions affecting cognition, such as dementia.