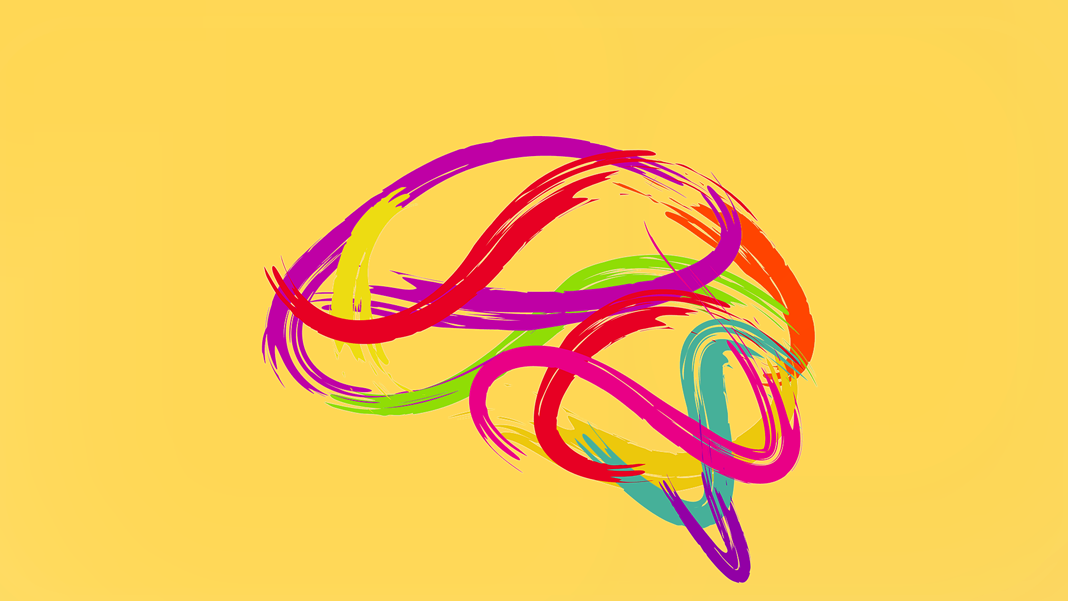
The brain is an exceptionally powerful computing machine, and AI researchers have long been trying to recreate its abilities. A team from IBM may have cracked the code with NorthPole, a fully digital chip that mimics the brain’s structure and efficiency.
When pitted against state-of-the-art graphics processing units-the chips most commonly used to run AI programs-IBM’s brain-like chip triumphed in several standard tests, while using up to 96 percent less energy.
From TrueNorth to SpiNNaker, they’ve spent a decade tapping into the brain’s architecture to better run AI algorithms.
Project to project, the goal has been the same: How can we build faster, more energy efficient chips that allow smaller devices-like our phones or computers in self-driving cars-to run AI on the “Edge.”
Edge computing can monitor and respond to problems in real-time without needing to send requests to remote server farms in the cloud.
Like switching from dial-up modems to fiber-optic internet, these chips could also speed up large AI models with minimal energy costs.
Traditional computer chips, in contrast, use digital processing-0s and 1s. If you’ve ever tried to convert an old VHS tape into a digital file, you’ll know it’s not a straightforward process. Most chips that mimic the brain use analog computing. Tightly packing 22 billion transistors onto 256 cores, the chip takes its cues from the brain by placing computing and memory modules next to each other.
The chip is especially relevant in light of increasingly costly, power-hungry AI models. The chip represents “Neural inference at the frontier of energy, space and time,” the authors wrote in their paper, published in Science.
Both creating the algorithms and running them requires massive amounts of computing power, resulting in high costs, processing delays, and a large carbon footprint. These popular AI models are loosely inspired by the brain’s inner workings.
They don’t mesh well with our current computers. The brain processes and stores memories in the same location.
One idea is to build analog computing chips similar to how the brain functions.
Rather than processing data using a system of discrete 0s and 1s-like on-or-off light switches-these chips function more like light dimmers. Because each computing “Node” can capture multiple states, this type of computing is faster and more energy efficient.
Analog chips also suffer from errors and noise. Although flexible and energy efficient, the chips are difficult to work with when processing large AI models.
The result is a stamp-sized chip that can beat the best GPUs in several standard tests. The team’s first step was to distribute data processing across multiple cores, while keeping memory and computing modules inside each core physically close.
Previous analog chips, like IBM’s TrueNorth, used a special material to combine computation and memory in one location.
Instead of going analog with non-standard materials, the NorthPole chip places standard memory and processing components next to each other.
The rest of NorthPole’s design borrows from the brain’s larger organization. The chip has a distributed array of cores like the cortex, the outermost layer of the brain responsible for sensing, reasoning, and decision-making.
Inspired by these communication channels, the team built two networks on the chip to democratize memory.
The team also developed software that cleverly delegates a problem in both space and time to each core-making sure no computing resources go to waste or collide with each other.
The software “Exploits the full capabilities of the architecture,” they explained in the paper, while helping integrate “Existing applications and workflows” into the chip.
IBM’s previous brain-inspired analog chip, NorthPole can support AI models that are 640 times larger, involving 3,000 times more computations.
The team next pitted NorthPole against several GPU chips in a series of performance tests.
The chip also processed data at lighting-fast speeds compared to GPUs on two difficult AI benchmark tests.
Some experts believe that Moore’s law-which posits that the number of transistors on a chip doubles every two years-is at death’s door. Although still in their infancy, alternative computing structures, such as brain-like hardware and quantum computing, are gaining steam.
Currently, there are 37 million transistors per square millimetre on the chip. Based on projections, the setup could easily expand to two billion, allowing larger algorithms to run on a single chip.